Replies: 1 comment 1 reply
-
@xoofee mixup typically helps on COCO for very large models that tend to overfit earlier. If your model is not overfitting there is no reason to introduce augmentaiton. |
Beta Was this translation helpful? Give feedback.
1 reply
Sign up for free
to join this conversation on GitHub.
Already have an account?
Sign in to comment
-
I train yolov5s on a subset of coco with
(1000 images per class and split 0.8/0.2 for train/val
I observe that
in case A it converges fast when mixup set to 0.0 , compared to 0.1 mixup. Mixup decreate mAP a little (5%)
in case B it converge in the same speed, how ever after 200 epochs, mAP with no mixup is better (21% v.s. 23%)
mAP@0.5 2 classes
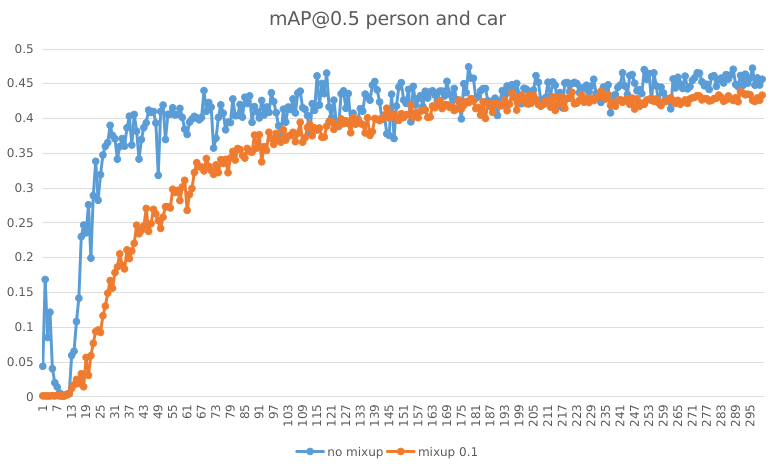
mAP@0.5 10 classes
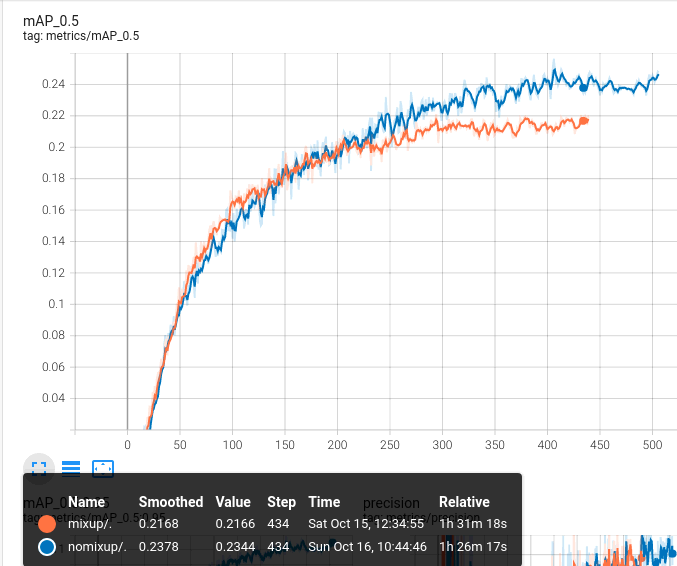
the only difference is value of mixup
lr0: 0.1
lrf: 0.1
momentum: 0.937
weight_decay: 0.0005
warmup_epochs: 3.0
warmup_momentum: 0.8
warmup_bias_lr: 0.1
box: 0.05
cls: 0.3
cls_pw: 1.0
obj: 0.7
obj_pw: 1.0
iou_t: 0.2
anchor_t: 4.0
fl_gamma: 0.0
hsv_h: 0.015
hsv_s: 0.7
hsv_v: 0.4
degrees: 0.0
translate: 0.1
scale: 0.9
shear: 0.0
perspective: 0.0
flipud: 0.0
fliplr: 0.5
mosaic: 1.0
mixup: 0.0
copy_paste: 0.1
Beta Was this translation helpful? Give feedback.
All reactions