-
Notifications
You must be signed in to change notification settings - Fork 1
/
Copy pathREADME.Rmd
547 lines (432 loc) · 16.8 KB
/
README.Rmd
1
2
3
4
5
6
7
8
9
10
11
12
13
14
15
16
17
18
19
20
21
22
23
24
25
26
27
28
29
30
31
32
33
34
35
36
37
38
39
40
41
42
43
44
45
46
47
48
49
50
51
52
53
54
55
56
57
58
59
60
61
62
63
64
65
66
67
68
69
70
71
72
73
74
75
76
77
78
79
80
81
82
83
84
85
86
87
88
89
90
91
92
93
94
95
96
97
98
99
100
101
102
103
104
105
106
107
108
109
110
111
112
113
114
115
116
117
118
119
120
121
122
123
124
125
126
127
128
129
130
131
132
133
134
135
136
137
138
139
140
141
142
143
144
145
146
147
148
149
150
151
152
153
154
155
156
157
158
159
160
161
162
163
164
165
166
167
168
169
170
171
172
173
174
175
176
177
178
179
180
181
182
183
184
185
186
187
188
189
190
191
192
193
194
195
196
197
198
199
200
201
202
203
204
205
206
207
208
209
210
211
212
213
214
215
216
217
218
219
220
221
222
223
224
225
226
227
228
229
230
231
232
233
234
235
236
237
238
239
240
241
242
243
244
245
246
247
248
249
250
251
252
253
254
255
256
257
258
259
260
261
262
263
264
265
266
267
268
269
270
271
272
273
274
275
276
277
278
279
280
281
282
283
284
285
286
287
288
289
290
291
292
293
294
295
296
297
298
299
300
301
302
303
304
305
306
307
308
309
310
311
312
313
314
315
316
317
318
319
320
321
322
323
324
325
326
327
328
329
330
331
332
333
334
335
336
337
338
339
340
341
342
343
344
345
346
347
348
349
350
351
352
353
354
355
356
357
358
359
360
361
362
363
364
365
366
367
368
369
370
371
372
373
374
375
376
377
378
379
380
381
382
383
384
385
386
387
388
389
390
391
392
393
394
395
396
397
398
399
400
401
402
403
404
405
406
407
408
409
410
411
412
413
414
415
416
417
418
419
420
421
422
423
424
425
426
427
428
429
430
431
432
433
434
435
436
437
438
439
440
441
442
443
444
445
446
447
448
449
450
451
452
453
454
455
456
457
458
459
460
461
462
463
464
465
466
467
468
469
470
471
472
473
474
475
476
477
478
479
480
481
482
483
484
485
486
487
488
489
490
491
492
493
494
495
496
497
498
499
500
501
502
503
504
505
506
507
508
509
510
511
512
513
514
515
516
517
518
519
520
521
522
523
524
525
526
527
528
529
530
531
532
533
534
535
536
537
538
539
540
541
542
543
544
545
546
547
---
output: github_document
---
<!-- README.md is generated from README.Rmd. Please edit that file -->
<!-- devtools::rmarkdown::render("README.Rmd") -->
<!-- Rscript -e "library(knitr); knit('README.Rmd')" -->
```{r, echo = FALSE}
knitr::opts_chunk$set(
collapse = TRUE,
comment = "#>",
fig.path = "README-"
)
```
# Mini `supersmartR` Workshop <img src="https://raw.githubusercontent.com/AntonelliLab/supersmartR/master/logo.png" height="300" align="right"/>
> Originally put together for a mini-workshop on 8 Nov. 2019 in Gothenburg,
Sweden.
* [`supersmartR`](https://github.com/AntonelliLab/supersmartR) is a series of
R packages that form a phylogenetic pipeline.
* The original [SUPERSMART](https://github.com/naturalis/supersmart) program
uses a "divide-and-conquer" apprach to constructing large phylogenetic trees.
* It combines both a supermatrix (an assembly of multiple gene/clusters into a
single matrix) and a supertree (merging multiple trees) approach.
* `supersmartR` packages are standalone packages with their own functions
and uses BUT they can be combined to recreate the supersmart pipeline.
### [SUPERSMART](http://www.supersmart-project.org/)

### [supersmartR](https://github.com/AntonelliLab/supersmartR)
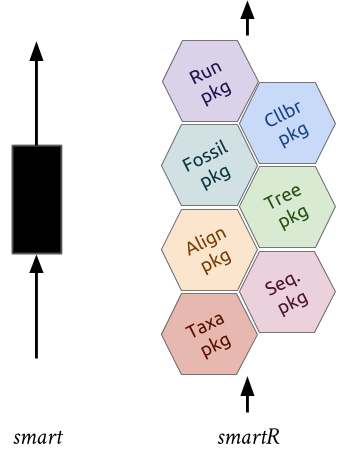
## The Workshop
This workshop we will [introduce the packages](#packages) and provide code to
run a simple [pipeline](#pipeline) to create a phylogenetic tree (a supertree
of all Guinea-pig-like species).
* [Introduction to `phylotaR`](#phylotar)
* [Introduction to `restez`](#restez)
* [Introduction to `outsider`](#outsider)
* [Introduction to `gaius`](#gaius)
* [Supertree pipeline](#pipeline)
**Target Duration ~1 hr**
* * *
# Prerequisites
* Software
* [R](https://cran.r-project.org/) (> 3.5)
* [RStudio](https://www.rstudio.com/)
* [Desktop Docker](https://docs.docker.com/install/) (Linux containers)
* Basic knowledge
* R
* Phylogenetics
(Windows users may struggle installing Docker Desktop, in which case
[Docker Toolbox](https://docs.docker.com/toolbox/toolbox_install_windows/) will
also work.)
### R packages
Install dependant packages.
```r
# remotes allows installation via GitHub
if (!'remotes' %in% installed.packages()) {
install.packages('remotes')
}
library(remotes)
# install latest outsider
install_github("antonellilab/outsider.base")
install_github("antonellilab/outsider")
# install latest restez
install_github("hannesmuehleisen/MonetDBLite-R")
install_github("ropensci/restez")
# install latest phylotaR
install_github("ropensci/phylotaR")
# install latest gaius
install_github("antonellilab/gaius")
```
If you're computer is set-up correctly with the R packages and Docker, you
should be able to run the below script without errors (don't worry about
security warnings).
```{r testoutsider, results='hold'}
## Code
library(outsider)
repo <- "dombennett/om..hello.world"
module_install(repo = repo, force = TRUE)
hello_world <- module_import(fname = "hello_world", repo = repo)
hello_world()
## Output
```
* * *
# Setup
To run all the code in this workshop you will need to:
* Download the zipped folder of this GitHub repo, [click here](https://github.com/AntonelliLab/supersmartR-workshop-mini/archive/master.zip).
* Unzip this folder and place it in a convenient location on your computer (e.g.
"my coding projects").
* Open RStudio and create a new project from this new folder.
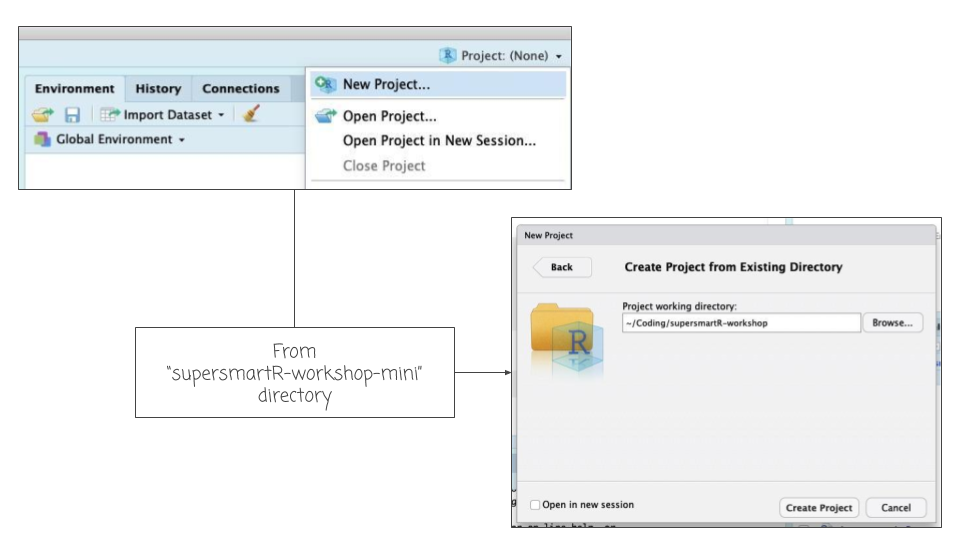
* * *
# Tutorials
## Packages
### [`phylotaR`](https://github.com/ropensci/phylotaR) <img src="https://raw.githubusercontent.com/ropensci/phylotaR/master/logo.png" height="200" align="right"/>
The `phylotaR` package downloads all sequences associated with a given
taxonomic group and then runs all-vs-all BLAST to identify clusters of
sequences suitable for phylogenetic analysis.
The process takes place across four stages:
* Taxise: identify taxonomic IDs
* Download: download sequences
* Cluster: run all-vs-all BLAST
* Cluser^2: run all-vs-all BLAST again
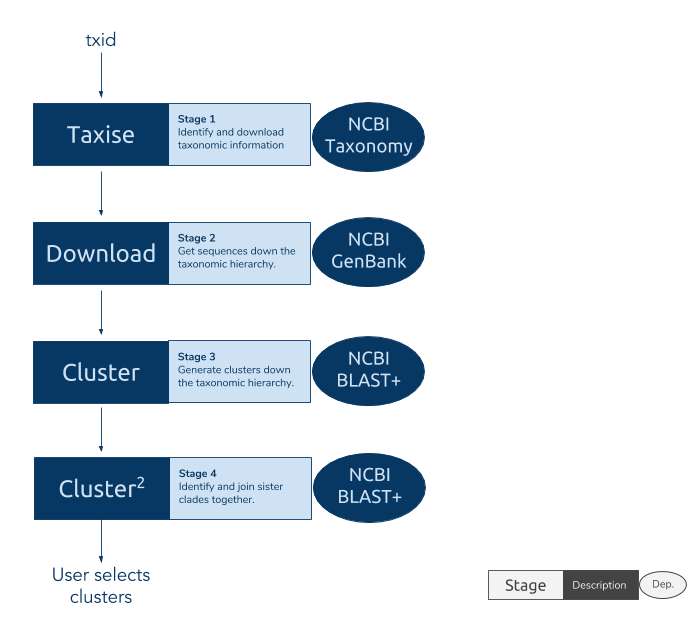
#### Setup
To run `phylotaR`, we need to set up a folder to host all downloaded files.
Parameters for setting up the folder are provided to the `setup` function.
```{r setup-phylotaR, include=TRUE, eval=FALSE}
# NOT RUN
library(phylotaR)
# available parameters
print(parameters())
# e.g. mnsql = 200 - minimum sequence length
# pass the parameters to the setup() function
# essential parmeters are: wd, txid
wd <- file.path(tempdir(), 'testing_phylotaR')
if (dir.exists(wd)) {
unlink(x = wd, recursive = TRUE, force = TRUE)
}
dir.create(wd)
setup(wd = wd, txid = '9504', outsider = TRUE, v = TRUE)
# to run the pipeline
# run(wd = wd)
```
#### Parsing results
Read in the phylotaR results with `read_phylota`. But here we will use the
pacakge example data, "Aotus".
##### Summarise the clusters
```{r phylotar-summary-results, echo=TRUE, results='hold'}
library(phylotaR)
data(aotus)
# generate summary stats for each cluster
smmry_tbl <- summary(aotus)
# Important details
# N_taxa - number of taxonomic entities associated with sequences in cluster
# N_seqs - number of sequences in cluster
# Med_sql - median sequence length of sequences in cluster
# MAD - measure of the deviation in sequence length of a cluster
# Definition - must common words in sequence definition lines
smmry_tbl[1:10, ]
```
```{r phylotar-summary-plot}
# plot
p <- plot_phylota_treemap(phylota = aotus, cids = aotus@cids[1:10],
area = 'nsq', fill = 'ntx')
print(p)
```
##### Understand the PhyLoTa table (`aotus`)
```{r phylota-table, results='hold'}
# CODE
# PhyLoTa table has ...
# clusters
aotus@clstrs
# sequences
aotus@sqs
# taxonomy
aotus@txdct
# A cluster is a list of sequences
aotus@clstrs@clstrs[[1]]
str(aotus@clstrs@clstrs[[1]])
# A sequence is a series of letters and associated metadata
aotus@sqs@sqs[[1]]
str(aotus@sqs@sqs[[1]])
# A taxonomic record is an ID and associated metadata
txid <- aotus@txids[[1]]
# Information can be extracted from ...
# clusters
get_clstr_slot(phylota = aotus, cid = aotus@cids[1:10], slt_nm = 'nsqs')
# sequences
get_sq_slot(phylota = aotus, sid = aotus@sids[1:10], slt_nm = 'nncltds')
# tax. records
get_tx_slot(phylota = aotus, txid = aotus@txids[1:10], slt_nm = 'scnm')
# Other useful convenience functions
get_nsqs(phylota = aotus, cid = aotus@cids[1:10])
get_ntaxa(phylota = aotus, cid = aotus@cids[1:10], rnk = 'species')
# OUTPUT
```
##### Select clusters
```{r phylotar-selection, results='hold'}
# CODE
# use the summary table to extract cids of interest
nrow(smmry_tbl)
# keep clusters with MAD above 0.5
smmry_tbl <- smmry_tbl[smmry_tbl[['MAD']] >= 0.5, ]
nrow(smmry_tbl)
# keep clusters with more than 10 seqs
smmry_tbl <- smmry_tbl[smmry_tbl[['N_seqs']] >= 10, ]
nrow(smmry_tbl)
# keep clusters with more than 4 species
nspp <- get_ntaxa(phylota = aotus, cid = smmry_tbl[['ID']], rnk = 'species')
selected_cids <- smmry_tbl[['ID']][nspp >= 4]
length(selected_cids)
# create selected PhyLoTa table
selected_clusters <- drop_clstrs(phylota = aotus, cid = selected_cids)
# OUTPUT
```
##### Plotting selected clusters
```{r phylota-plotting, results='hold'}
# CODE
# extract scientific names for taxonomic IDs
scnms <- get_tx_slot(phylota = selected_clusters, txid = selected_clusters@txids,
slt_nm = 'scnm')
# plot presence/absence
plot_phylota_pa(phylota = selected_clusters, cids = selected_clusters@cids,
txids = selected_clusters@txids, txnms = scnms)
```
##### Writing to file
```{r phylota-writeout, results='hold'}
# CODE
# reduce clusters to repr. of 1 seq. per sp.
reduced_clusters <- drop_by_rank(phylota = selected_clusters, rnk = 'species',
n = 1)
reduced_clusters
# write out first cluster
sids <- reduced_clusters@clstrs[['0']]@sids
txids <- get_txids(phylota = reduced_clusters, sid = sids, rnk = 'species')
scnms <- get_tx_slot(phylota = reduced_clusters, txid = txids, slt_nm = 'scnm')
outfile <- file.path(tempdir(), 'cluster_1.fasta')
write_sqs(phylota = reduced_clusters, sid = sids, sq_nm = scnms,
outfile = outfile)
cat(readLines(outfile), sep = '\n')
# OUTPUT
```
### [`restez`](https://github.com/ropensci/restez) <img src="https://raw.githubusercontent.com/ropensci/restez/master/logo.png" height="200" align="right"/>
`restez` is a package that allows users to download whole chunks of NCBI's
[GenBank](https://www.ncbi.nlm.nih.gov/genbank/). The package works by:
* Downloading compressed files of sections of GenBank
* Unpacking these files and building a local GenBank copy
* Providing generic functions for interacting with the local copy
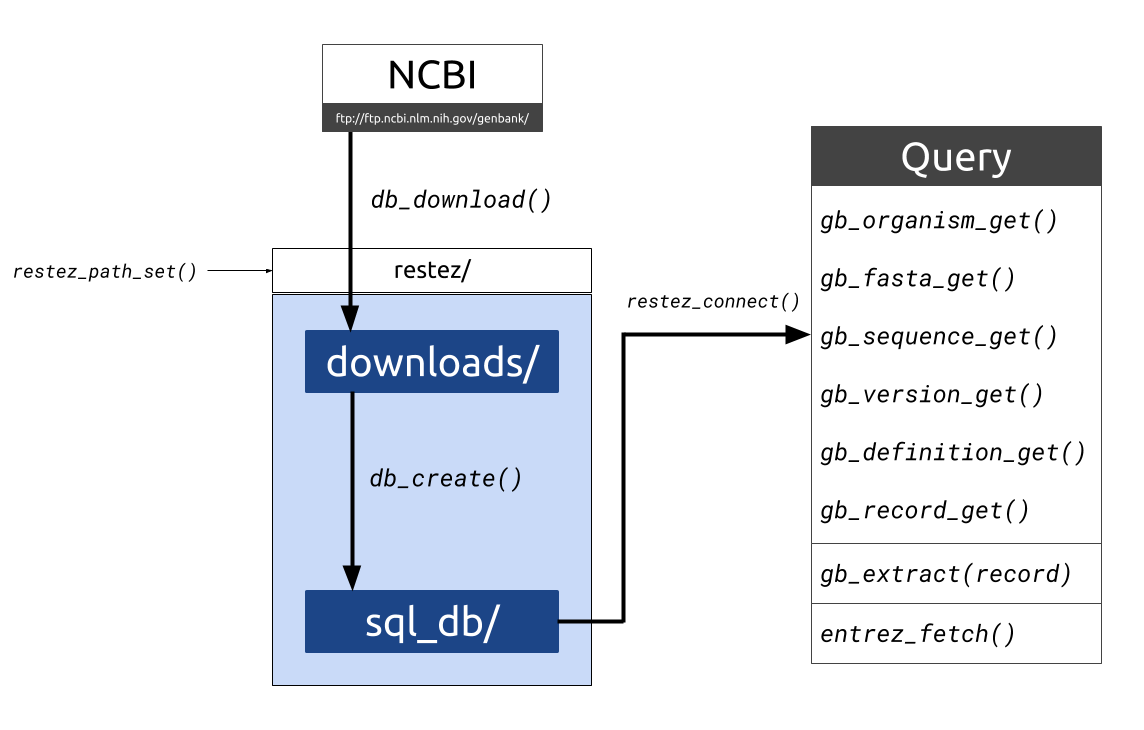
#### Set-up your first `restez` database
Here, we will do the following:
1. Specify a location for our database (`restez_path`)
2. Download the smallest section of GenBank (unannotated)
3. Build a local database
```{r delete-database, include=FALSE}
rstz_pth <- file.path(tempdir(), 'unannotated_database')
if (dir.exists(rstz_pth)) {
unlink(x = rstz_pth, recursive = TRUE, force = TRUE)
}
```
```{r restez-setup, echo=TRUE, results='hold'}
# CODE
library(restez)
# 1. Set the filepath for where the database will be stored
rstz_pth <- file.path(tempdir(), 'unannotated_database')
if (!dir.exists(rstz_pth)) {
dir.create(rstz_pth)
}
restez_path_set(filepath = rstz_pth)
# 2. Download
# select number 20, for unannoated (the smallest section)
db_download(preselection = '20')
# 3. Create database
# connect to empty database
restez_connect()
db_create()
# always disconnect from a database when not in use.
restez_disconnect()
# OUTPUT
```
#### Query the database
We can send queries to the database using two different methods: `restez`
functions or [`rentrez`](https://ropensci.org/tutorials/rentrez_tutorial/)
wrappers.
> **What is `rentrez`?** The `rentrez` package allows users to interact with
NCBI Entrez. `restez` wraps around some of its functions so that instead of
sending queries across the internet, the local database is checked first.
```{r querying, echo=TRUE, results='hold'}
# CODE
# import library, point to database and connect
library(restez)
rstz_pth <- file.path(tempdir(), 'unannotated_database')
restez_path_set(filepath = rstz_pth)
restez_connect()
# Check the status
restez_status()
# Get a random ID from the database
id <- sample(x = list_db_ids(n = 100), size = 1)
# print record information
record <- gb_record_get(id)
cat(record)
# see ?gb_record_get for more query functions
# always disconnect
restez_disconnect()
# OUTPUT
```
### [`outsider`](https://github.com/antonellilab/outsider) <img src="https://raw.githubusercontent.com/antonellilab/outsider/master/logo.png" height="200" align="right"/>
`outsider` is a package that allows users to install and run external code
within the R environment. This is very useful when trying to construct
pipelines that make use of a variety of code. `outsider` requires Docker to
work. It should be able to launch any (?!) command-line program.
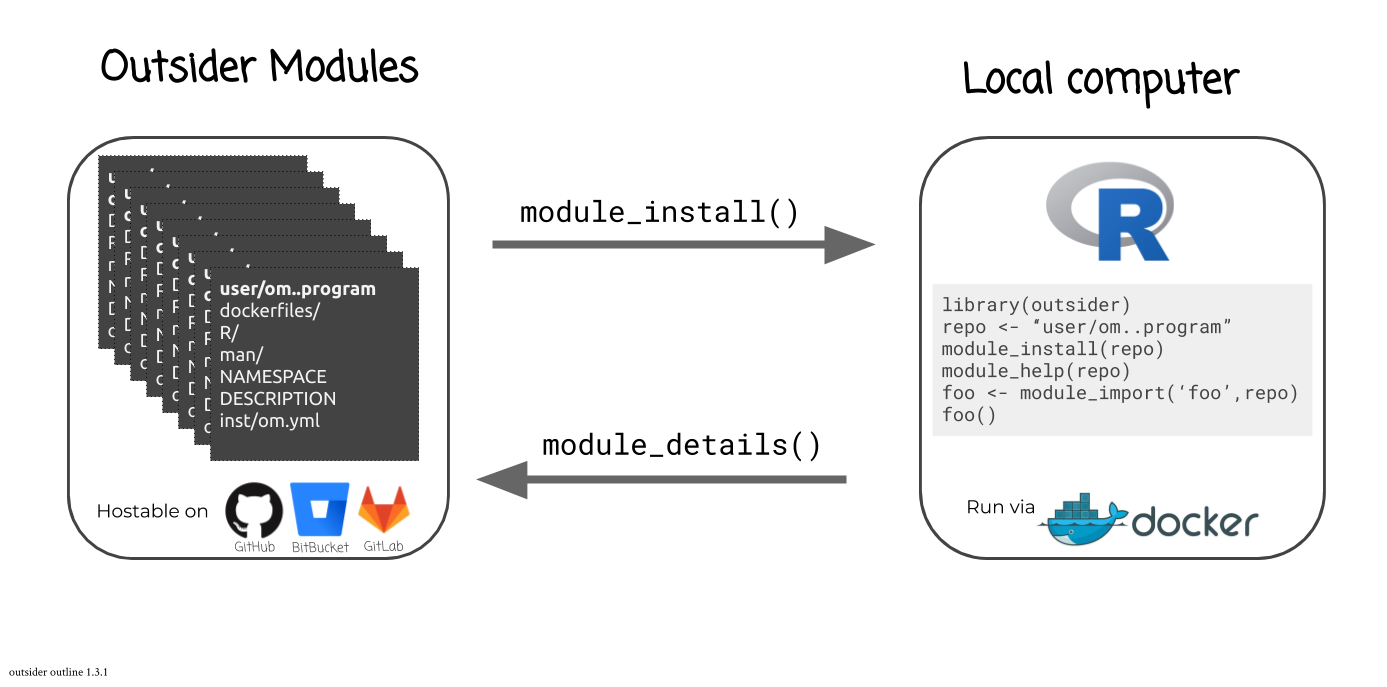
You can find out what modules are available for a given coding-service using:
```{r outsider-available, echo=TRUE, results='hold'}
library(outsider)
module_details(service = 'github')
```
There is also a package called
["outsider.devtools"](https://github.com/AntonelliLab/outsider.devtools) that
makes it easier to create your own moduules, see `other/outsider_devtools.R`
#### Running alignment software
To demonstrate, let's run an alignment software tool,
[mafft](https://mafft.cbrc.jp/alignment/software/), from within R. Let's
install a module for mafft, and then run it on some test sequences
("ex_seqs.fasta").
#### Install
```{r remove-mafft, include=FALSE}
if (outsider::is_module_installed('dombennett/om..mafft')) {
outsider::module_uninstall('dombennett/om..mafft')
}
```
```{r outsider-mafft, echo=TRUE, results='hold'}
# CODE
library(outsider)
# squelch text to console
verbosity_set(show_program = FALSE, show_docker = FALSE)
# github repo to where the module is located
repo <- 'dombennett/om..mafft'
# install mafft
module_install(repo = repo, force = TRUE)
# look up available functions
(module_functions(repo = repo))
# import mafft function
mafft <- module_import(fname = 'mafft', repo = repo)
# test function
mafft(arglist = '--help')
# OUTPUT
```
#### Align
```{r outsider-align, echo=TRUE, results='hold'}
library(outsider)
# Use example mafft nucleotide data
ex_seqs_file <- file.path(getwd(), 'data', 'ex_seqs.fasta')
(file.exists(ex_seqs_file))
# Run
mafft <- module_import(fname = 'mafft', repo = 'dombennett/om..mafft')
ex_al_file <- file.path(getwd(), 'data', "ex_al.fasta")
mafft(arglist = c('--auto', ex_seqs_file, '>', ex_al_file))
(file.exists(ex_al_file))
# View alignment
cat(readLines(con = ex_al_file, n = 50), sep = '\n')
```
### [`gaius`](https://github.com/antonellilab/gaius) <img src="https://raw.githubusercontent.com/antonellilab/gaius/master/logo.png" height="200" align="right"/>
* `gaius` acts as the nexus package for the SUPERSMART pipeline in R
* It imports both alignments and trees and produces supermatrices and
supertrees
* Its main role is to identify monophyletic groups of a given number of taxa
for species-level trees
* The main "trick" is to use a pre-existing tree
* It can do a few clever things: there can be any number of levels of backbone,
backbone supermatrices are constucted from an assortment of the best
monophyletic sequences
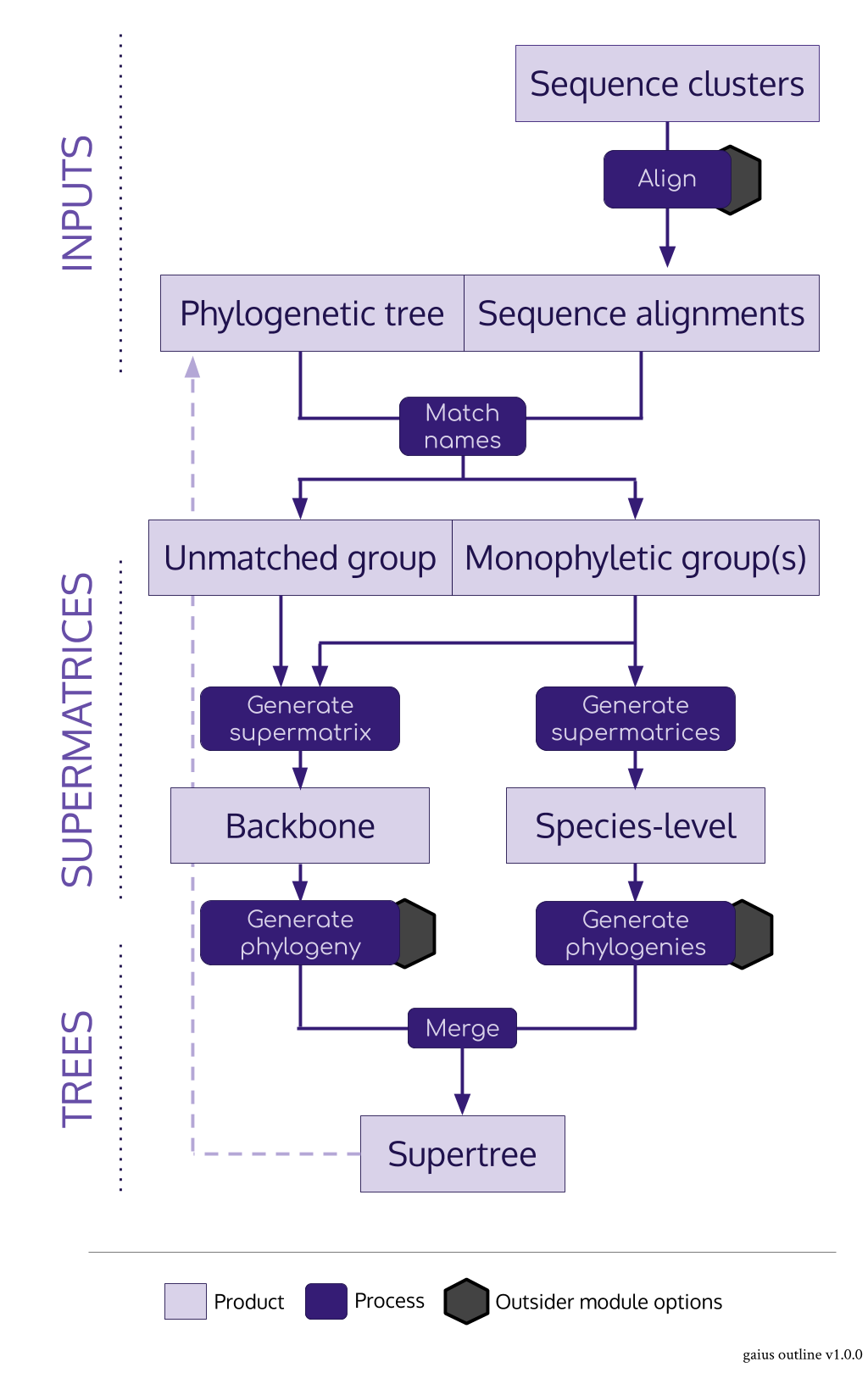
```{r gaius, results='hold'}
# CODE
library(gaius)
# vars
alignment_dir <- file.path(getwd(), 'data', 'gaius_alignments')
tree_file <- file.path(getwd(), 'data', 'taxtree.tre')
# alignment files
alignment_files <- file.path(alignment_dir,
list.files(path = alignment_dir,
pattern = '.fasta'))
(alignment_files[1:2])
# get alignment names
alignment_names <- names_from_alignments(alignment_files)
(alignment_names[1:10])
# tree tip names
tree_names <- names_from_tree(tree_file)
(tree_names[1:10])
# match alignment names to those in tree
matched_names <- name_match(alignment_names = alignment_names,
tree_names = tree_names)
(matched_names)
# identify monophyletic groups
groups <- groups_get(tree_file = tree_file, matched_names = matched_names)
(groups)
# read in alignments
alignment_list <- alignment_read(flpths = alignment_files)
(alignment_list)
# ^ for a better idea of the alignment, try ...
# viz(alignment_list[[1]])
# construct supermatrices
supermatrices <- supermatrices_get(alignment_list = alignment_list,
groups = groups, min_ngenes = 2,
min_ntips = 3, min_nbps = 100,
column_cutoff = 0, tip_cutoff = 0.1)
(supermatrices)
# OUTPUT
```
## Pipeline
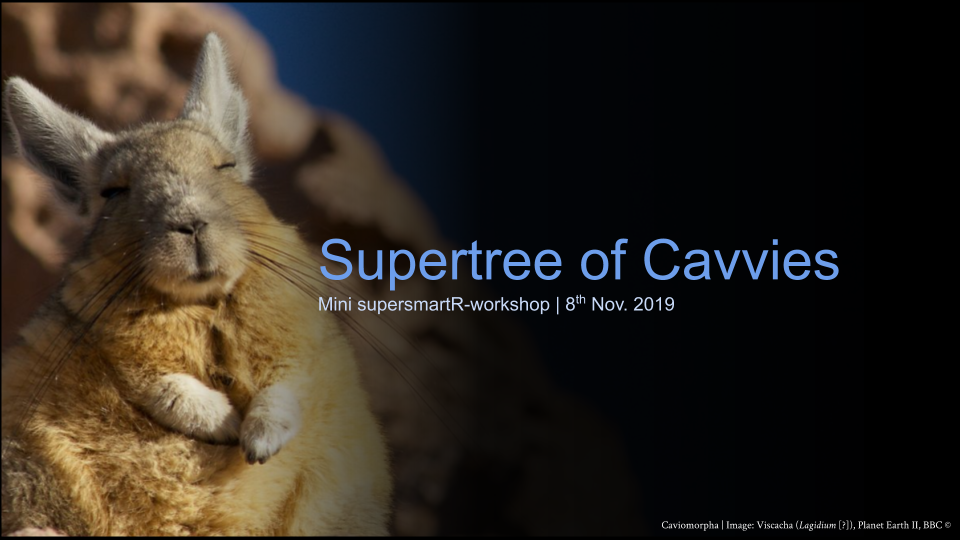
A complete pipeline for constructing a phylogenetic tree of all Caviomorpha
species is contained in `pipeline/`. The pipeline uses the above packages and their functions. We can run each script of the pipeline using `source()`. (To
save time we will skip the `restez` and `phylotaR` steps and download a
completed folder of the first part of the results.)
```{r pipeline, results='hold'}
## Code
# minor outsider set-up
if (file.exists('gc_setup.R')) {
source(file = 'gc_setup.R', local = FALSE, echo = FALSE, print.eval = FALSE)
} else {
threads <- 4
}
# save time by skipping the first two stages by using the ready
# 1_phylotaR/ in data/
zipfile <- file.path('data', '1_phylotaR.zip')
if (file.exists(zipfile)) {
utils::unzip(zipfile = zipfile, exdir = 'pipeline', overwrite = TRUE)
}
# run each script in the pipeline
stage_scripts <- c('2_clusters.R', '3_align.R', '4_supermatrix.R',
'5_phylogeny.R', '6_supertree.R', '7_view.R')
start_time <- Sys.time()
cat('Running pipeline ....\n', sep = '')
for (stage_script in stage_scripts) {
cat('... ', crayon::green(stage_script), '\n', sep = '')
scriptenv <- new.env()
scriptenv$threads <- threads
suppressMessages(source(file.path('pipeline', stage_script),
local = scriptenv, echo = FALSE, print.eval = FALSE))
}
end_time <- Sys.time()
duration <- difftime(end_time, start_time, units = 'mins')
cat('Duration: ', crayon::red(round(x = duration, digits = 3)), ' minutes.\n')
# clear-up
outsider::ssh_teardown()
## Output
```
The resulting tree consists of 185 tips, generating from 16 gene/clusters.

***
**Learn more: [`supersmartR` GitHub Page](https://github.com/AntonelliLab/supersmartR)**